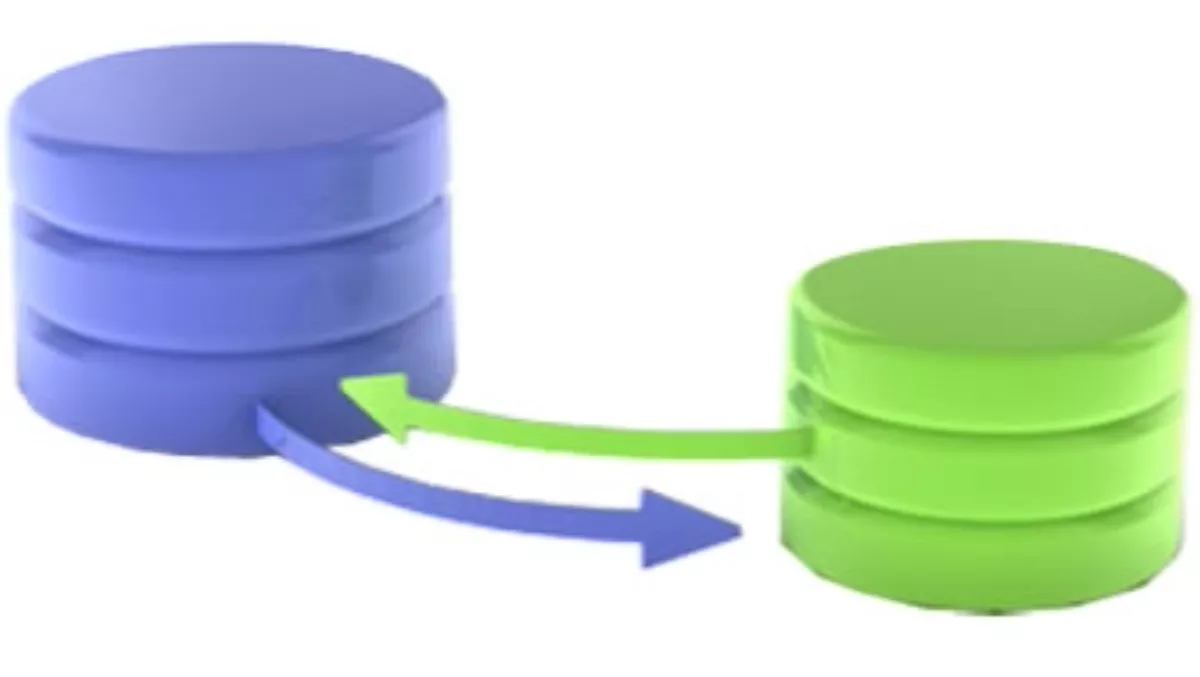
Avoiding the five pitfalls of data migration
According to Informatica senior consultant, Clive Astbury, data migration is often the lynchpin of a larger initiative in which an organisation invests strategy, budget, and time. "Execution requires retiring legacy applications and implementing new ones to support the new approach to business. The program will not succeed if delivery of the data is delayed or if the data does not correctly support the business." There are some simple rules however, Astbury says, that should ensure data migtation goes smoothly 1. Failure to follow best practices. Many IT teams assume they can apply the same best practices to application data migrations as they do to application code development. Although this approach seems logical, it leads to delays and failure because the deliverable for data migration is unlike that of any other IT project. For application code development, the goal is to deliver code that adds new functionality or improves processes. For application data migration, the goal is to deliver a production-ready set of data for a new application. This key difference must be taken into account as the project is structured. The application data migration team should understand which practices are distinct to the project, including team structure, risk mitigation, data discovery, legacy retirement, agility, audit and validation, mock loads, production data, data quality, and cutover. 2. Skipping data discovery. If the team that understood an organisations' old mainframe data has long since retired, the systems have grown organically over many years, or an organisation is trying to merge data from an acquisition, there may be no repository of information about the legacy applications and the data, which can cause real problems in a data migration project. A data steward misidentifying the actual source of data is not uncommon. Rarely does the actual data content look anything like the team is expecting. Data stewards and other users tend to see data only in an extracted, aggregated, or presentational form. Data migration development teams, who work with granular, table-level data, can also be challenged in knowing what data meets business needs. Although the data model, relationships, column names, and structures help provide the metadata necessary for a good understanding of data, they rarely offer the full picture. For an application data migration, it's better for data discoveries, and their surprises, to occur primarily at the beginning of the project. If data analysis occurs up front, the need for costly extract and mapping rework is minmised, and so are potential delays. To avoid the impacts of not understanding the data that are caused by skipping or minimising data discovery, the team should engage in master data discovery, data profiling and conduct a target impact analysis. 3. Incomplete data movement strategy. Moving business-critical data needs to be done purposefully and carefully as this data runs the organisation. Many teams assume that a data migration is a simple mapping of data from one table to another. Business users will likely demand audits of the move, there will almost always be challenges about the quality of the data, and there will be frequent project changes and data discoveries that can create substantial delays. If data isn't moved in a way that ensures that it supports the new application, organisations operations can be adversely affected. Data migration is risky, and the pitfall is not realising how to mitigate that risk during the move. The main areas to focus on that will ensure the application data migration team move data that works are reusability, data quality, audit and validation, and connectivity. 4. Lack of collaboration. Most data migration teams do not recognise the value of collaborating with the business users and the data stewards. Business users are the data experts that are able to make necessary decisions about when data is 'good enough', what needs to be fixed at the source, what needs to be enhanced and how, and which transformations are working properly. They determine what data to pull forward and what to archive, respond to data quality scorecards and approve data quality fixes. Data migration projects that succeed and come in on time and on budget with the full confidence of end users involve business users from start to finish. 5. Inadequate tools. Too many teams either attempt to hand code a data migration or do not use the tools that they have effectively. Each data migration is unique and every business imperative is different, but certain common needs cut across all industries and all types of applications. Choosing the right tools and knowing how to use them dramatically improve the odds of success for every application data migration. Astbury says, "Implementing the appropriate tools and processes means that the common pitfalls of data migration can be avoided."