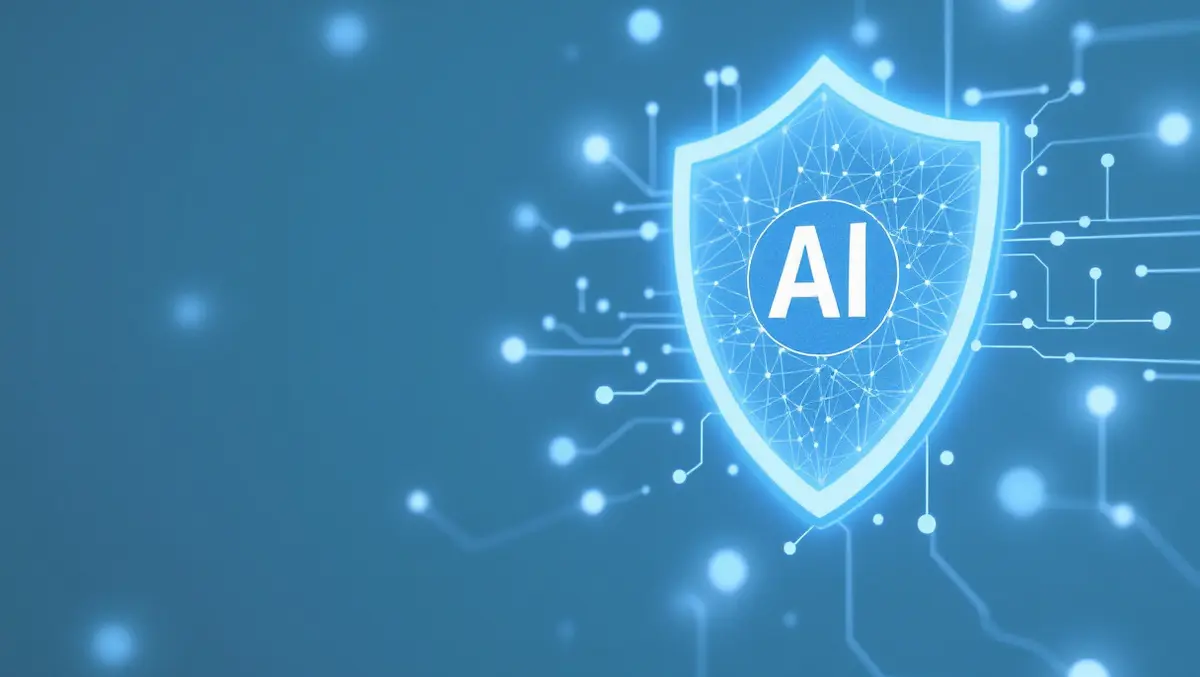
Google DeepMind reveals new strategy to defend Gemini 2.5 AI
Google DeepMind has published a new white paper detailing security measures implemented in its Gemini 2.5 large language model family to address indirect prompt injection attacks.
Indirect prompt injection poses a cybersecurity issue for artificial intelligence systems, in which malicious instructions can be hidden within user data, such as emails or documents, and interpreted by language models as legitimate commands. The company describes these attacks as a challenge, requiring models to distinguish between genuine user intentions and potentially manipulative embedded content.
The Security and Privacy Research team at Google DeepMind noted in its white paper, Lessons from Defending Gemini Against Indirect Prompt Injections, that as language models access increasing amounts of user data and external information, they become attractive targets for such attacks. The team outlined a strategic blueprint for mitigating these threats and increasing the resilience of its AI systems.
The researchers state the objective is to develop not only capable, but secure AI agents, saying, "Our commitment to build not just capable, but secure AI agents, means we're continually working to understand how Gemini might respond to indirect prompt injections and make it more resilient against them."
Manual efforts to identify vulnerabilities in models were described as time-consuming and inefficient, especially given the rapid evolution of large language models. As a result, Google DeepMind built an automated system designed to continually probe Gemini's defences to identify and address weaknesses more effectively.
An integral part of the approach is automated red teaming (ART), where internal teams simulate realistic attacks on the Gemini model. Through ART, previously unknown potential weaknesses can be discovered and addressed. "A core part of our security strategy is automated red teaming (ART), where our internal Gemini team constantly attacks Gemini in realistic ways to uncover potential security weaknesses in the model. Using this technique, among other efforts detailed in our white paper, has helped significantly increase Gemini's protection rate against indirect prompt injection attacks during tool-use, making Gemini 2.5 our most secure model family to date," the research team stated.
In their evaluation, the team assessed several defence strategies from the wider research community in addition to their own methods. Initial results indicated that baseline mitigations were effective against basic, non-adaptive attacks, with a significant reduction in success rates for such intrusions. However, when facing more sophisticated and adaptive attacks, these methods proved less successful. According to the white paper, "Baseline mitigations showed promise against basic, non-adaptive attacks, significantly reducing the attack success rate. However, malicious actors increasingly use adaptive attacks that are specifically designed to evolve and adapt with ART to circumvent the defence being tested."
The findings highlighted that defences tested only against static attacks could provide a misleading sense of protection. The team observed, "Successful baseline defences like Spotlighting or Self-reflection became much less effective against adaptive attacks learning how to deal with and bypass static defence approaches. This finding illustrates a key point: relying on defences tested only against static attacks offers a false sense of security. For robust security, it is critical to evaluate adaptive attacks that evolve in response to potential defences."
To increase intrinsic resilience, DeepMind has implemented a process termed 'model hardening'. The organisation fine-tuned Gemini on a dataset containing scenarios of indirect prompt injections generated by ART, teaching the model to disregard embedded malicious instructions and focus on authentic user requests.
"We fine-tuned Gemini on a large dataset of realistic scenarios, where ART generates effective indirect prompt injections targeting sensitive information. This taught Gemini to ignore the malicious embedded instruction and follow the original user request, thereby only providing the correct, safe response it should give. This allows the model to innately understand how to handle compromised information that evolves over time as part of adaptive attacks," Google DeepMind's Security and Privacy Research team stated.
The researchers report that this strategy has lowered the attack success rate without substantially affecting performance on standard tasks. They noted, "This model hardening has significantly boosted Gemini's ability to identify and ignore injected instructions, lowering its attack success rate. And importantly, without significantly impacting the model's performance on normal tasks."
The team cautioned that no security system is completely impervious, stating, "It's important to note that even with model hardening, no model is completely immune. Determined attackers might still find new vulnerabilities. Therefore, our goal is to make attacks much harder, costlier, and more complex for adversaries."
DeepMind's methodology involves a multi-layered, 'defence-in-depth' approach, combining model hardening, input and output analysis, and systemic guardrails. "Protecting AI models against attacks like indirect prompt injections requires 'defence-in-depth' – using multiple layers of protection, including model hardening, input/output checks (like classifiers), and system-level guardrails. Combating indirect prompt injections is a key way we're implementing our agentic security principles and guidelines to develop agents responsibly," the team stated.
Google DeepMind underlines that ongoing and adaptive evaluations, continuous improvement of defences, and building resilience into the models are necessary to address evolving security threats in advanced AI systems. "Securing advanced AI systems against specific, evolving threats like indirect prompt injection is an ongoing process. It demands pursuing continuous and adaptive evaluation, improving existing defences and exploring new ones, and building inherent resilience into the models themselves. By layering defences and learning constantly, we can enable AI assistants like Gemini to continue to be both incredibly helpful and trustworthy."