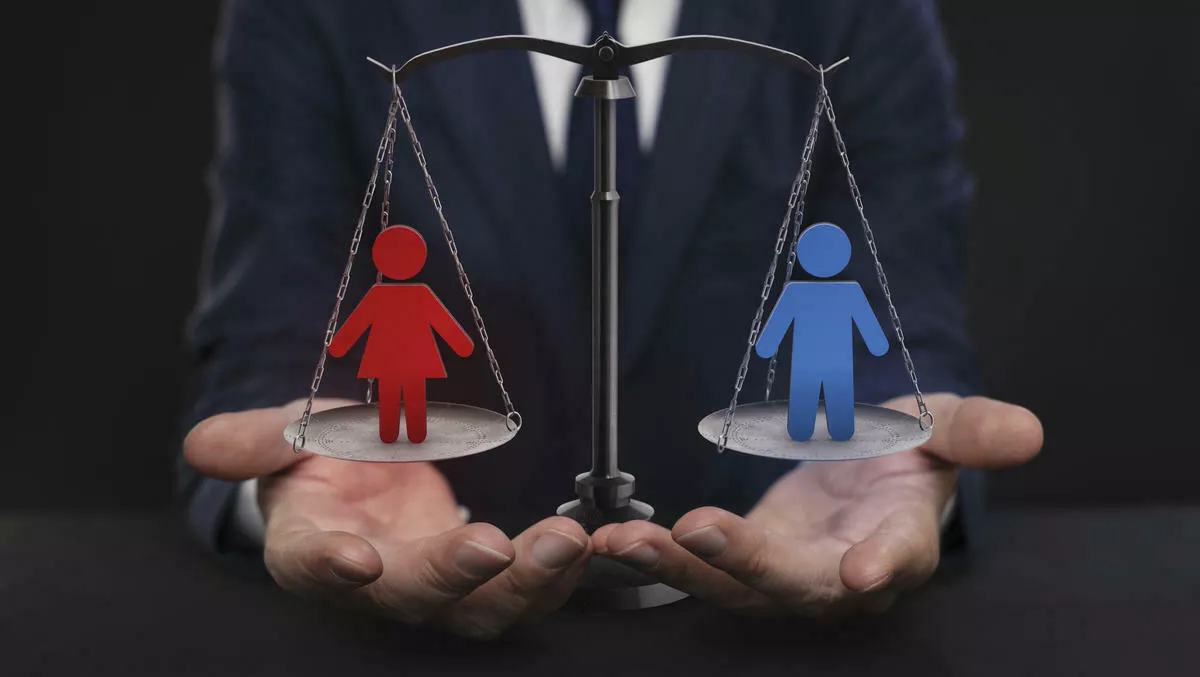
How mathematics can limit gender bias in AI & ML
Three major universities have released a new research paper that outlines how machine learning designers can safeguard their creations against the undesirable effects of racial and gender bias.
Researchers from Stanford University, the University of Massachusetts Amherst and the Universidade Federal do Rio Grande do Sol published a paper called Preventing undesirable behavior of intelligent machines.
The paper explains that mathematical criteria could allow a machine learning platform to train artificial intelligence (AI) to avoid unwanted outcomes.
If 'unsafe' or 'unfair' outcomes can be defined in terms of mathematics, then it could be possible to create algorithms that learn how to avoid these outcomes.
"We want to advance AI that respects the values of its human users and justifies the trust we place in autonomous systems," explains Stanford assistant professor of computer science and senior author of the paper, Emma Brunskill.
The researchers also wanted to develop a set of techniques that would make it easy for users to specify what sorts of unwanted behaviour they want to constrain and enable machine learning designers to predict with confidence that a system trained using past data can be relied upon when it is applied in real-world circumstances.
THEY TESTED THEIR THEORIES BY TRYING TO IMPROVE THE FAIRNESS of algorithms that predict GPAs of college students based on exam results, which researchers say is a common practice that can result in gender bias. As a result of the testing, the algorithm exhibited less synthetic gender bias than existing methods.
According to researchers, existing methods have no inbuilt fairness filter, or the algorithms designed to achieve fairness are too limited in scope.
"We show how the designers of machine learning algorithms can make it easier for people who want to build AI into their products and services to describe unwanted outcomes or behaviours that the AI system will avoid with high-probability," says University of Massachusetts Amherst assistant professor of computer science and first author of the paper, Philip Thomas.
The researchers propose that a 'Seldonian framework' will make it easier for machine learning designers to build behaviour-avoidance instructions into all sorts of algorithms, in a way that can enable them to assess the probability that trained systems will function properly in the real world.
"Thinking about how we can create algorithms that best respect values like safety and fairness is essential as society increasingly relies on AI," Brunskill concludes.
The research was supported in part by Adobe, the US National Science Foundation and the US Institute of Educational Sciences.