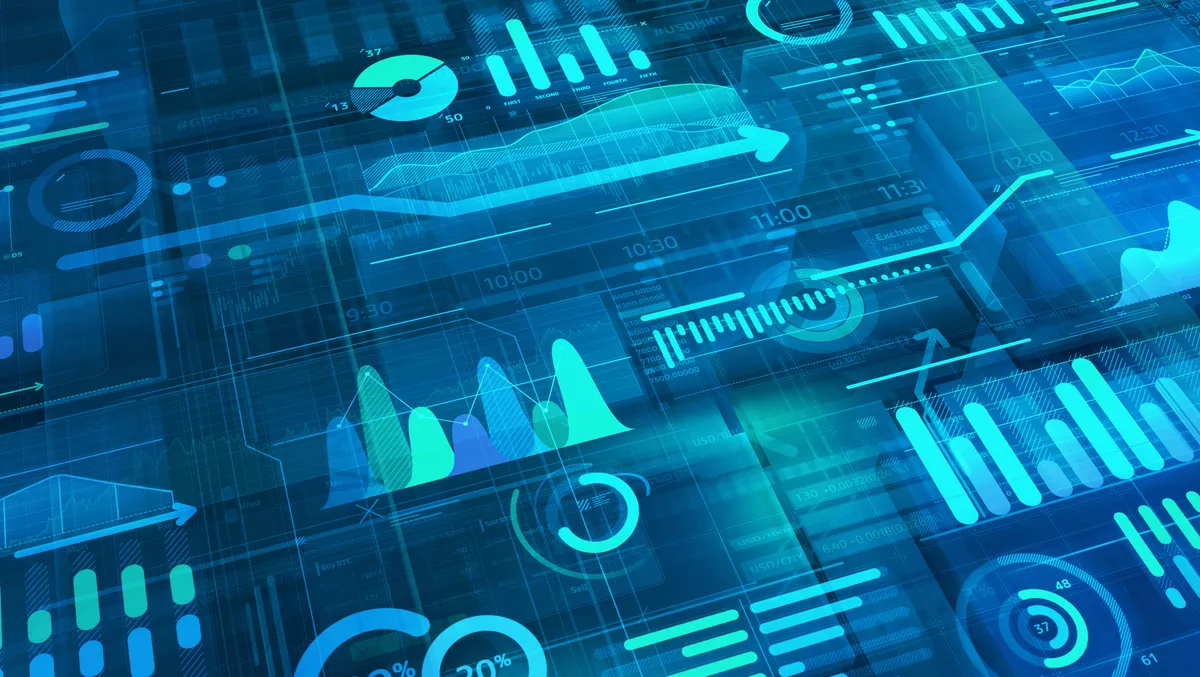
Turning big data into value with the right technology
Digital transformation has become so common in business that many businesses are upgrading or adopting new technology without having a clear understanding of the value it can deliver, according to SNP.
The company says the key to the success of any transformation project tends to lie in the data that these new technologies can use.
With the right data, organisations can transform not just their technology but their entire operating model and unique selling proposition. However, turning data into business value requires organisations to have the right technology in place to extract insights from that data.
"When organisations take the path of least resistance or choose solutions purely based on cost, they can miss out on the potentially revolutionary capabilities that would let them turn data into business value quickly and relatively easily," says Brent Paterson, managing director, Australia and New Zealand, SNP.
"For example, when moving to SAP S/4HANA, organisations can gain significant value from the platform's data analytics capabilities but, to do so, it's essential to set the expectations clearly upfront in the project," he says.
"This can determine the type of implementation the business chooses, which will in turn affect costs. However, it will also dramatically affect the return on investment that the organisation achieves as a result of the implementation, so it's worth spending some time on due diligence before setting a migration plan in play.
According to SNP, in order to gain maximum value from transformational projects, organisations must understand what measurable results they want to see from the initiative so they can determine what kind of data and analytics should be used. They must start with the end in mind or risk a failed project. Above all, they should focus on maximising the organisation's ability to extract value from data.
There are four ways organisations can maximise the value of their data:
1. Data storage strategies
Data storage strategies must include ways to easily access and work with that data. Corporate data lakes provide one way to aggregate data from various systems to deliver a more comprehensive, overarching view of data that can help drive data-driven operations and decisions. Examples include predictive analytics that let businesses make smarter decisions, faster, as well as Internet of Things (IoT) applications.
2. Data archiving and decommissioning of legacy systems
Data has a shelf life and there comes a time when it needs to be archived. Legacy systems may need to be decommissioned, especially in the case of a merger or acquisition or a system upgrade or transformation. Data can't simply be deleted; it usually needs to be retained for a set period under legislation. Therefore, organisations need to ensure they're moving outdated data to a data lake. This way, the organisation can still access and manage that data without incurring high costs associated with keeping legacy systems running.
3. Data security and privacy
Digital transformation projects can open up data to the risk of being lost or hacked. It's essential to put data protection measures in place to safeguard data during the transformation process. Data migrations can create considerable costs and risks, especially in the context of a merger or acquisition, or divestment. Data handovers and migrations must be managed properly to avoid data privacy issues that can trigger contract penalties or put the company in breach of the Privacy Act.
4. End-to-end data lifecycle management
As organisations work with different types of data across the entire organisational ecosystem, the vast volume of data can quickly spiral out of control. This can lead to risks in terms of data privacy, access, and governance. It's essential to manage the end-to-end data lifecycle with a solution that covers archiving, management, and decommissioning of data to maintain compliance and maximise the value of the data that is used to drive decision-making.
Automating data migration and management processes can help reduce risk and make data more accessible for decision-making and transformation projects. Businesses need to understand how to use data for analytics using innovative data science applications such as machine learning and artificial intelligence so they can maximise the value of their data.
"Managing data effectively takes a clear strategic approach and the right technology," says Paterson.
"Applying automation where possible can help minimise the burden on organisations while they leverage data for business value," he says.
"It's important to choose a solution that reduces complexity along with a provider that is an expert in managing data transformation projects that help organisations reach their ideal outcomes.