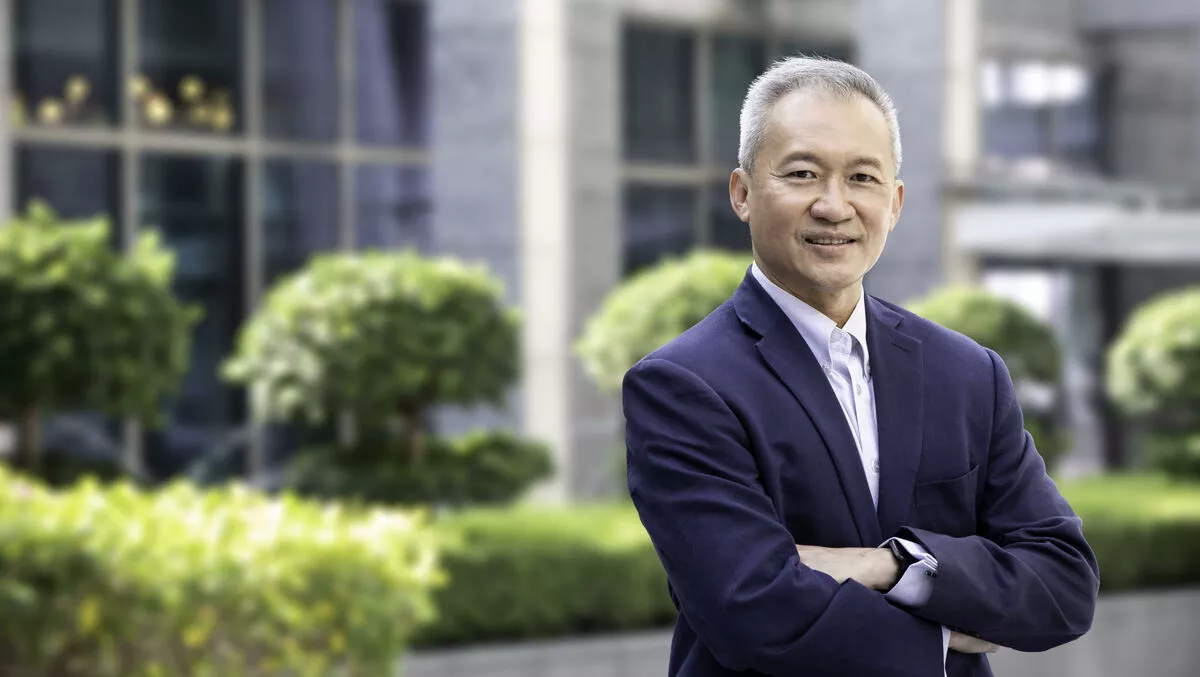
Uncovering hidden eCommerce, marketing gems through knowledge graphs
Marketers have access to more data than ever before - and it's growing exponentially. The pandemic-driven acceleration in digitisation has created vast repositories of data around consumers, products and purchases. According to Statista, global data creation - the total amount of data created, captured, copied, and consumed - is projected to grow to more than 180 zettabytes by 2025 globally.
The challenge is how to sift through it and identify the trends and patterns that can be used to generate valuable insights. As a massive stream of data, it has little to no value to marketers without context and relevance. How can marketers transform realms of information and make data meaningful and useful?
The power of knowledge graphs
In today's data-rich world, marketers can convert data into greater marketing intelligence through knowledge graphs. Unlike a traditional database in table form, with rows and columns of data, knowledge graphs store data as linked nodes and the relationships between them. This data can then be displayed visually, as colours and shapes, making it easy to detect patterns and anomalies.
For example, in an Excel spreadsheet you can fairly easily log one data set (customers) against another (their purchase history). But trying to add in additional context such as demographic information, the path to purchase, time and date of purchase, and weather patterns in a locality is difficult, unwieldy, and impossible to do in a single sheet. However, this context can be critical for spotting patterns of who buys what, when, and how.
Knowledge graphs are designed to uncover insights into customer needs, product, and market trends, alleviating the challenges of continually growing, highly interconnected datasets. They excel in connecting and managing masses of buyer and product data to answer complex queries.
Analysing marketing effectiveness
For example, let's say we want to analyse website activity and track how people are finding different pages on a website: for example, through clicking an advert, via a search engine, from social media, or in an email link. This will help us understand if a current marketing campaign is effective and provide insight into the behaviour of site visitors who become customers.
In a knowledge graph, all the pages and marketing channels become nodes linked to one another. By adding context to the links (relationships), we can start to make complex queries. For example, if we have data about visit times, we can analyse the evolution of individual marketing channel performance and interest in specific pages. Then by adding data about visitor location, we can analyse if certain channels work better for certain geographic markets.
Real-time recommendations
Real-time recommendation engines are critical for online retailers. The aim is to flash up relevant product suggestions and invite shoppers to add last-minute extras to an online cart. This benefits both sides: the vendor can offer high-margin items, overstocks and promotions, while the purchaser discovers useful and relevant items, improving their customer experience.
To generate relevant recommendations involves instantly correlating product, customer, inventory, supplier, logistics and even social sentiment data, as well as instantly capturing any new interests shown in the customer's current visit. Sifting through all this data in real-time is beyond the ability of a conventional relational database. However, matching historical and session data is trivial for a knowledge graph.
A knowledge graph can also use and weight multiple recommendation methods, such as recommending based on similar users or products, on user history and profile, or on business strategy (promotions, margin, inventory).
Knowing your customers
In the post-cookie world, knowledge graphs provide a way to connect huge amounts of buyer and product data to generate insights into product trends and customer needs. Knowledge graphs are much faster at doing this than traditional means. They can be used to analyse web traffic and clickstream data and build unique customer profiles.
US media conglomerate Meredith Corporation used graph algorithms to transform billions of page views into millions of pseudonymous identifiers with rich browsing profiles. It then consolidated 350 million profiles that would have been considered unique individuals with different interests and patterns into 163 million richer and more accurate profiles. This has enabled it to understand customers and market better.
Gartner predicts that by 2025, graph technologies will be used in 80% of data and analytics innovations, up from 10% this year. Ultimately, knowledge graphs empower marketers to achieve their targets and drive competitive edge and overall success for the business.