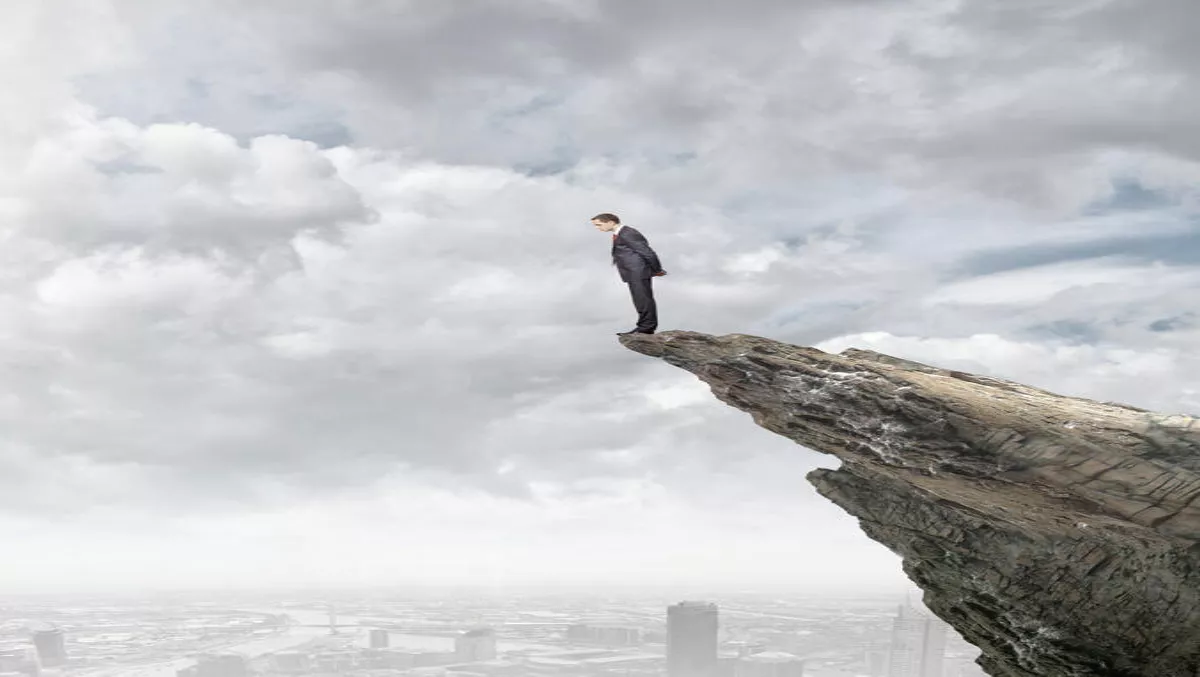
Accenture: Industrial Internet of Things, big data & analytics at the edge
The Internet of Things (IoT) has led to an unprecedented level of connectivity within enterprise.
According to Cisco, as many as 50 billion devices could be connected to the Internet of Things (IoT) by 2020, including numerous industrial processes.
The Industrial Internet of Things (IIoT) has facilitated connectivity between manufacturing machines, industrial equipment, pipelines and other remote devices which, in turn, has created vast amounts of operational data.
Although big data is a valuable resource for organisations, many businesses are stuck in the "what, how, where" phase of their IIoT thinking because of the challenges of implementation.
Enterprises must connect, identify and secure numerous different devices, machines, and appliances. They must capture, transmit and store data from those things.
They must analyse and learn from that data, and integrate it into core operating and information systems. They must educate customers, employees and partners in its use. And they must rethink business processes and measures of success.
But there is a way forward.
There is an approach that can quickly generate tangible and measurable value from the IIoT. It is an approach that means deploying advanced analytics solutions "at the edge.
Analytics at the edge is a new and different solution. It involves carrying out conventional big data analysis but moving more of it to the edge of the network. In other words, closer to the "essence" or the "thing" that is the source of the new data, whether that be a vehicle, industrial machine, fitness device or washing machine.
This involves running the data through an analytics algorithm as it is created, at the edge of a corporate network.
The process sorts information in real-time and only saves useful information that is worth saving for later use. Edge Analytics moves away from the principle in Big Data Analytics that "the more data the better".
Pushing as much computing workload as close to the edge as possible can bring serious benefits, particularly where communication costs are high or where instant action is needed. Edge Analytics can drive business value through:
Improving equipment up time and efficiency
Failure in a subsystem or component, or the impact of running a component in a degraded state, can be predicted in real time (and continually refined as more data is analysed) and used to inform operational use and maintenance scheduling.
Automated "self-correcting" actions that continuously optimise performance can also be triggered.
Reducing maintenance costs
Equipment failure can be avoided through preventative maintenance undertaken when actually needed, rather than at fixed periods in a predetermined schedule.
More repairs can be carried out on first visits by giving mechanics detailed instructions about the causes of a problem, what action is needed, and what parts are required.
Lowering spares inventory
Maintenance crews have an earlier and more precise visibility into future failures and breakdowns, making a spares inventory vastly more efficient.
Take the hypothetical failure in one part of a manufacturing system. An analytics algorithm interpreting the data at the network edge can automatically shut down the faulty machine and simultaneously send an alert to personnel so the part could be replaced.
But today's edge capabilities are still relatively unsophisticated, lacking anything like the computing power that cloud services can provide.
New approaches, therefore, need to be found.
The challenge in pushing computation closer to these edge devices is that their capabilities are dictated by their environment.
Unlike Big Data Analytics, Edge Analytics doesn't require large numbers of servers, computing power for machine learning and advanced analytics.
But they must have a small processing footprint. Take a Fitbit fitness device, it operates as an individual sensor with little processing capability that can send data to the cloud for computation.
The answer to this challenge is to design, build and deploy analytical models that address specific problems or objectives. In the process, new value-added capabilities can emerge.
Edge Analytics could be used to optimise uptime and maintenance for an individual oil well, for example, while Big Data cloud-based models work across multiple locations at an enterprise level.
The benefits of edge analytics' problem-focused approach will initially be seen at the edge of the network. That means optimisation will first occur at the level of individual pieces of equipment.
Realising the wider benefits of edge capabilities enterprise-wide will require analysis and optimisation further up the chain.
For example, the management of individual industrial equipment parts with edge capabilities will maximise the uptime and utilisation of each piece of equipment, but optimising parts management and maintenance scheduling across an entire fleet is a different story.
It means aggregating all the individual equipment outputs and then applying more traditional forms of analytics. Most organisations will thus opt for a hybrid analytics approach, incorporating both edge and cloud capabilities, optimised for their individual requirements and circumstances.
Starting at the edge and working towards the centre is the best place to start.
Edge capabilities represent the first step in an organisation's journey to capture the immense value that lies in the billions of connected devices set to join the IIoT.