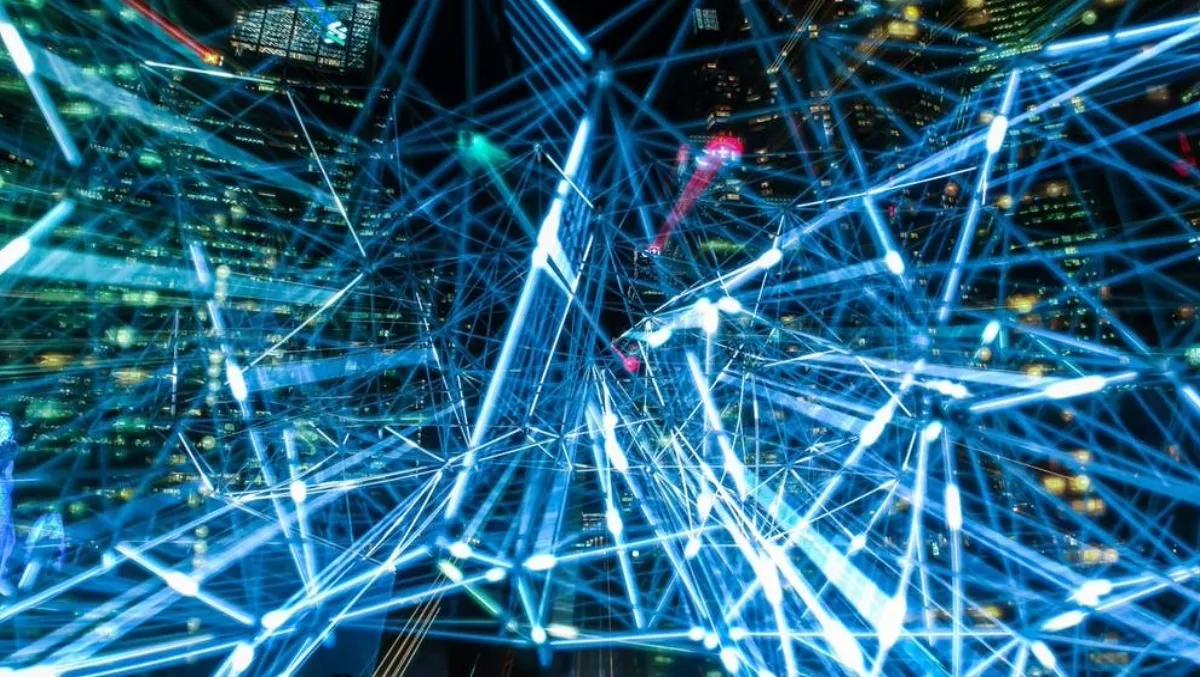
For many years now, experts have been calling artificial intelligence (AI) the next frontier in technology, and recently, we have seen those predictions come to light for consumers.
From Siri to Alexa, AI has manifested itself in a range of consumer-facing products that aim to assist and supplement the menial tasks of daily life. Just think: when was the last time you asked Siri to give you the temperature outside, to set a reminder, or to order a product from your favourite retailer?
Halfway through 2018, the conversation around AI is shifting to the enterprise, as companies worldwide are beginning to adapt AI as a means to enhance employee experience and remain agile in today's technological environment.
However, the stakes for the tasks being carried out by AI in the enterprise are often much higher – and come with significantly greater risks. As such, enterprise AI must be adopted quite differently than consumer AI. Alexa can easily tell you the depth of the deepest point in the Pacific Ocean with little consequence, but would you trust Alexa to carry out a multi-million dollar deal involving operations or a product rollout seamlessly – and without human intervention? While the technology may be capable, the risk is often too large to take.
Yet, integrating AI into enterprises is imminent, and those businesses that fail to embrace this type of technology risk becoming extinct. The question is no longer whether to use AI in business, but rather, which specific tasks are appropriate for automating and what are the best practices for making it most valuable for your business?
Perhaps the most obvious starting point for enterprise AI usage is data storage and retrieval.
Creating and maintaining seamlessness in software solutions is no easy task, but it can be made fundamentally easier with AI. This is where the importance of data comes in – and becomes part of the technological solution.
For example, many of our day-to-day technologies face constant upgrades that require support from IT teams. With AI and Big Data, this process – which takes up a significant amount of an IT team members' time – can now be fully automated. Additionally, updates to the data itself can now be handled by technology, rather than by an employee.
The ability to capture data from across your enterprise—whether generated by disparate applications, people, or IoT infrastructure–offers tremendous potential. The value of AI is completely driven by the breadth and quality of the data. A data center or data warehouse stores data that has been generated for a specific purpose, in a specific format, in files or folders. Layering services on top of a separate repository are no longer enough. The best solutions will allow for data ingestion and training off a data lake.
Data lakes store data in its organic format, with no hierarchy. This enables the complex integration points to prepare, cleanse, or even update data points.
Data lakes are built on strong foundations centred around metadata. A metadata-driven approach to storing and consuming information forms a key part of decision-making as the business is able to search, catalogue, and marshal data to help deliver on heterogeneous integration requirements, ad-hoc reporting and referencing needs, and networking collections of data.
Insights and investments are infinitely scalable by ingesting more content to make better-informed business decisions. Data lakes can improve your analytics profiles and provide increasingly rich data sets to build more powerful machine learning processes.
By using data lakes to store their data, an enterprise can put their toe in the water, figuratively speaking, and make use of the benefits AI has to offer.
By Infor SVP and GM Helen Masters.