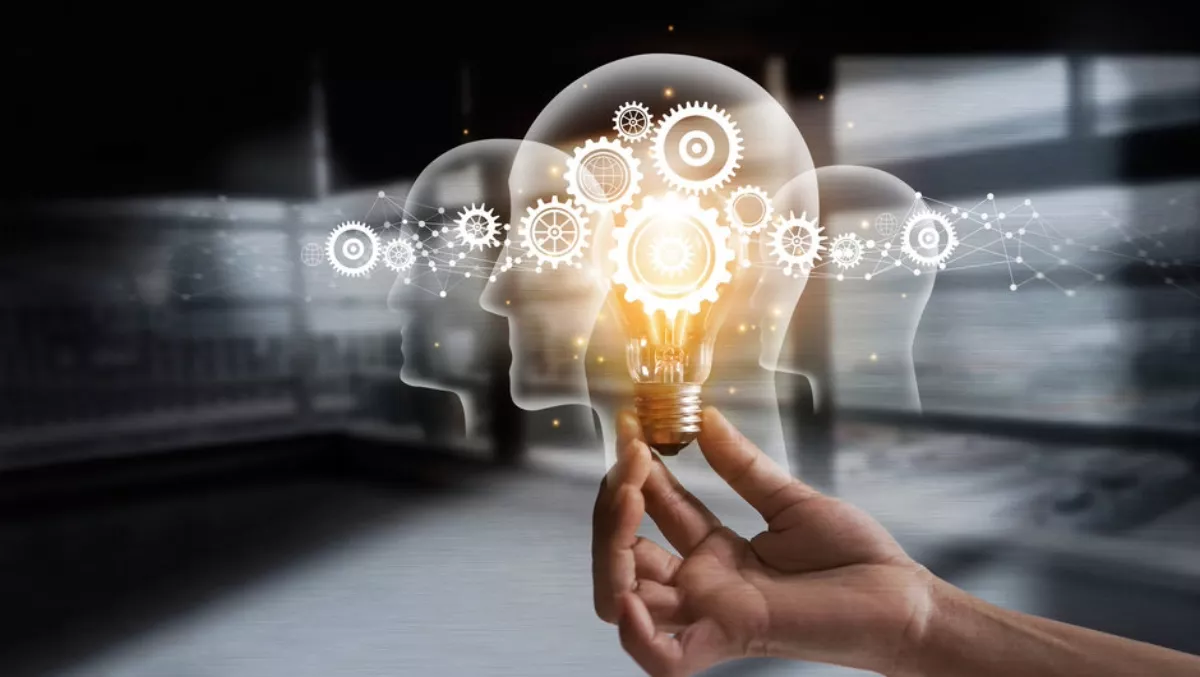
AI: Get started now, but on the right foot.
The speculation around Artificial Intelligence (AI) will continue to escalate in 2019. The hype will occasionally teeter between visions of apocalyptic machine-domination and utopian worlds where humans no longer need to work - although both extremes are still science fiction.
While AI is going to gain widespread adoption in the coming year, the applications will primarily be proof-of-concept test cases. Focused experimentation is a necessary prerequisite - and smart investment to make - before full implementation.
The current state of AI
Nearly 60% of the companies with more than $1 billion in revenue have at least pilot AI programmes underway, says a report from consulting firm McKinsey. In addition, Forrester Research estimates that revenue from AI-related software will nearly triple to $2.9 billion over the next three years. And by 2021, robotic automation technology will be doing the equivalent work of nearly 4.3 million humans worldwide.
While robotics is often the top-of-mind application associated with AI technology, the use cases are far more varied and typically behind the scenes.
Today, AI is successfully being used in search engines, predictive analytics, chatbots, and voice-activated personal assistants like Alexa. It's being embedded in software solutions for managing facility resources, supporting diversity, optimising inventory, sales forecasting, and anticipating customers' next purchases, among the many use cases. Industries like healthcare and manufacturing, driven by needs for efficiency, are spurring further innovation.
Despite the proven benefits, mass adoption is still in the future, with some challenges to be addressed.
Top three speed bumps slowing down progress
1. Early examples
Many of the early test cases revolve around retail and consumer applications. Business applications have been slower to materialise. The examples are limited and narrow-focused, often unique to a single company, product category or niche vertical. It's hard to learn from examples that are totally unrelated to your own market landscape.
2. Best practices yet to be defined
Another obstacle to full-scale deployment is the current lack of well-defined best practices. The documentation of early-adopter deployments are often sketches, deliberately vague to protect proprietary concepts. Companies will need to do their own research rather than count on implementation how-to details being readily available in the public domain.
3. Unknown financial impact
Limited understanding of financial impact is another hurdle yet to be crossed. The successful applications garnering press coverage, so far, often have focused on technology, driven by IT teams, not line-of-business managers with specific market pains to fix or quantifiable goals to reach. This technology-centric approach has value, but limitations, such as lack of data to support a business case or return on investment (ROI) story.
2019 is the year to build the business case and collect quantifiable results, which can be used to present large-scale proposals and gain support from stakeholders - especially gatekeepers for funding.
Three benefits the experimentation stage will yield
1. Cost justification
Through a phase of experimentation, organisations will discover what works, and what doesn't - and the bottom-line implications, which can be found from increased productivity, reduction of waste, boosts in customer spending, or new revenue streams. The bigger, the better, but even small gains over time can provide the necessary cost justification.
2. Scalable model
Besides generating RoI data, a well-documented test case can also provide the scalable framework for future expansion of AI adoption. A discovery decision-making tool, coaching services, process automation, and built-in intelligent workflows are some of the ways innovative solution providers are helping customers quickly roll out pilot projects. The exploration process can be as short as two months when the right tools are used.
3. Team buy-in
A successful test case that involves a positive experience for users will help overcome team fears of being replaced or marginalised by AI. Proactively demonstrating to the workforce that AI will be a tool, not a replacement, will help overcome scepticism that can derail projects. By engaging people at multiple levels in the organisation, the pilot project can help build a new mindset - one that fully embraces AI and its vast potential.
Conclusion
This strategic planning and budget setting season is the time to set expectations for AI initiatives and allocate resources. While a big-bang project may be the long-term goal, it's important to remember that test flights must occur first. This means budgets for a discovery phase, time for planning methodology, and flexibility to adjust the plan if needed.
Choosing to work with a solutions provider or implementation services provider that can provide support tools will help ramp up the process smoothly and avoid common mistakes.
In summation: Get started now, but on the right foot.