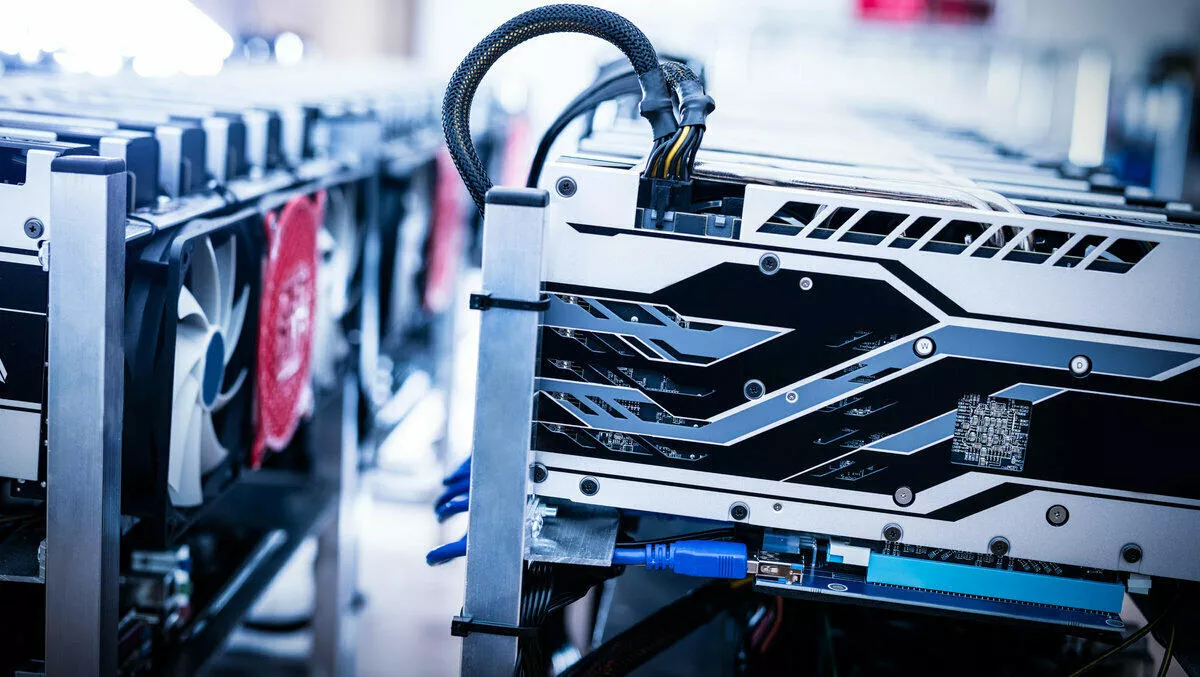
NVIDIA's fascinating role in artificial intelligence
On the 30th of September 2012, something significant changed in the world of computing. A computer programme was able to accurately identify the contents of over 14 million images.
That year the winner of the ImageNet challenge, was a system called AlexNet with an accuracy of around 85%. That was at least 10% better than the runner up.
What made this artificial intelligence (AI) breakthrough possible was the use of graphical processing units (GPUs) to accelerate the machine learning (ML).
This heralded a significant opportunity for the manufacturer of these GPUs, NVIDIA.
The GPU cards they had long sold to gamers now had a significant new application: accelerating machine learning (ML) and AI.
NVIDIA in 2006 had launched its CUDA software library and API to enable alternative uses of its cards, but the ImageNet competition win in 2012 brought GPU usage for AI into the mainstream.
Nowadays, researchers in many fields use the companies' GPUs, as well as all the major cloud service providers that offer NVIDIA GPU instances for AI/ML workloads. These include Alibaba Cloud, Amazon Web Services, Google Cloud, IBM Cloud, Microsoft Azure and Oracle Cloud.
"The NVIDIA platform provides end-to-end development of AI from data processing and the training of AI models to deployment in applications," says NVIDIA's Director of Product Management and Marketing, Paresh Kharya.
"For AI model training, the NVIDIA platform essentially provides a time machine by dramatically reducing the training time. This helps data scientists and researchers iterate faster to create new AI models and solve many different types of use cases. AI models that would take weeks or months with alternatives can now be trained in minutes and hours with NVIDIA products," says Kharya.
"When these AI models are deployed in applications like conversational AI, understanding video, and providing recommendations, the NVIDIA platform enables real-time processing and efficiently serves them to billions of users," says Kharya.
The use of GPUs to accelerate AI - ML workloads can make a huge difference - often well over 100 times faster, depending upon a lot of different factors. Other compute-intensive applications such as science and cryptography, can benefit from GPU usage.
The company has also been hard at work fine-tuning its hardware, with the introduction of specialised cores for TensorFlow, which is a commonly used open source library in ML.
When we look back to uses like mining cryptocurrency, in the end the limitations boiled down to energy usage. NVIDIA has been hard at work on this area also:
"NVIDIA GPUs enable the world's most energy-efficient solutions for AI, ML and HPC applications. Innovations like Tensor Cores in NVIDIA GPUs deliver unprecedented levels of performance needed for AI with extreme energy efficiency. Together with our ecosystem, we're very focused on energy efficiency at all levels of design -- from processor design to systems, supercomputers, and full-scale data centers. NVIDIA GPUs are 20-25 times more energy efficient than traditional CPU servers for AI workloads. As a result, NVIDIA GPUs power 23 of the top 25 most energy-efficient supercomputer systems in the world as measured by the Green 500 list," says Kharya.
See the Green 500 Supercomputer list here.
In addition to the CUDA software libraries, NVIDIA also has VGrid and VComputeServer tools that help to run and distribute these huge GPU workloads across a bunch of servers and GPU cards.
"With vComputeServer, IT admins can better streamline management of GPU-accelerated virtualised servers while retaining existing workflows and lowering overall operational costs. Compared to CPU-only servers, vComputeServer with four NVIDIA V100 GPUs accelerates deep learning 50x faster, delivering performance near bare metal," says NVIDIA Senior Director Product Marketing for GRID, Anne Hecht.
In summary
Artificial intelligence use is expanding across our lives, and this is generating massive new workloads for both the initial research as well as in deployment. NVIDIA GPUs have a pivotal role in accelerating these workloads. Coupled with its cloud partners, the cost to use NVIDIA GPUs has never been more accessible.